SapienzaNLP @ AAAI 2022
First AI conference of 2022!
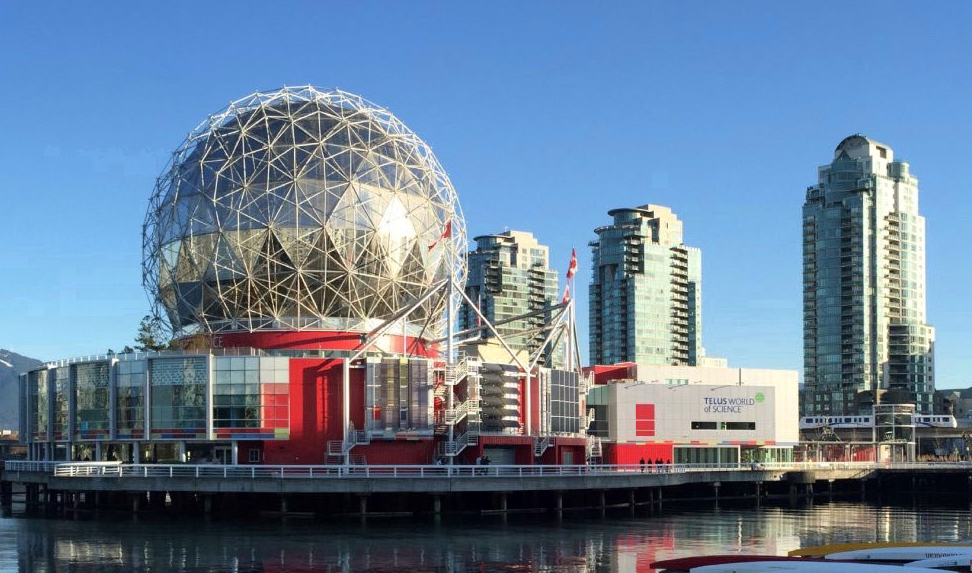
The Sapienza NLP group is proud to present two new papers at AAAI 2022! This time, we will showcase our work on semantic typing of events and visual definition modeling. Not only that, we will also introduce our "blue sky" idea on BabelNet Meaning Representation (BMR).
Our main conference papers are:
- Visual Definition Modeling: Challenging Vision & Language Models to Define Words and Objects
- STEPS: Semantic Typing of Event Processes with a Sequence-to-Sequence Approach
Plus, a "blue sky" idea:
- BabelNet Meaning Representation: A Fully Semantic Formalism to Overcome Language Barriers
Visual Definition Modeling: Challenging Vision & Language Models to Define Words and Objects
by B. Scarlini, T. Pasini, R. Navigli
Architectures that model language and vision together have received much attention in recent years. Nonetheless, most tasks in this field focus on end-to-end applications without providing insights on whether it is the underlying semantics of visual objects or words that is captured. In this paper we draw on the established Definition Modeling paradigm and enhance it by grounding, for the first time, textual definitions to visual representations. We name this new task Visual Definition Modeling and put forward DEMETER and DIONYSUS, two benchmarks where, given an image as context, models have to generate a textual definition for a target being either 1) a word that describes the image, or 2) an object patch therein. To measure the difficulty of our tasks we finetuned six different baselines and analyzed their performances, which show that a text-only encoder-decoder model is more effective than models pretrained for handling inputs of both modalities concurrently. This demonstrates the complexity of our benchmarks and encourages more research on text generation conditioned on multimodal inputs. The datasets for both benchmarks are available at https://github.com/SapienzaNLP/visual-definition-modeling as well as the code to reproduce our models.
STEPS: Semantic Typing of Event Processes with a Sequence-to-Sequence Approach
by S. Pepe, E. Barba, R. Blloshmi, R. Navigli
Enabling computers to comprehend the intent of human actions by processing language is one of the fundamental goals of Natural Language Understanding. An emerging task in this context is that of free-form event process typing, which aims at understanding the overall goal of a protagonist in terms of an action and an object, given a sequence of events. This task was initially treated as a learning-to-rank problem by exploiting the similarity between processes and action/object textual definitions. However, this approach appears to be overly complex, binds the output types to a fixed inventory for possible word definitions and, moreover, leaves space for further enhancements as regards performance. In this paper, we advance the field by reformulating the free-form event process typing task as a sequence generation problem and put forward STEPS, an end-to-end approach for producing user intent in terms of actions and objects only, dispensing with the need for their definitions. In addition to this, we eliminate several dataset constraints set by previous works, while at the same time significantly outperforming them. We release the data and software at https://github.com/SapienzaNLP/steps.
BabelNet Meaning Representation: A Fully Semantic Formalism to Overcome Language Barriers
by R. Navigli, R. Blloshmi, A. C. Martinez Lorenzo
Conceptual representations of meaning have long been the general focus of Artificial Intelligence (AI) towards the fundamental goal of machine understanding, with innumerable efforts made in Knowledge Representation, Speech and Natural Language Processing, Computer Vision, inter alia. Even today, at the core of Natural Language Understanding lies the task of Semantic Parsing, the objective of which is to convert natural sentences into machine-readable representations. Through this paper, we aim to revamp the historical dream of AI, by putting forward a novel, all-embracing, fully semantic meaning representation, that goes beyond the many existing formalisms. Indeed, we tackle their key limits by fully abstracting text into meaning and introducing language-independent concepts and semantic relations, in order to obtain an interlingual representation. Our proposal aims to overcome the language barrier, and connect not only texts across languages, but also images, videos, speech and sound, and logical formulas, across many fields of AI.